How We Got Here
The Evolution of Generative AI
Harrison Kirby
10/31/20244 min read
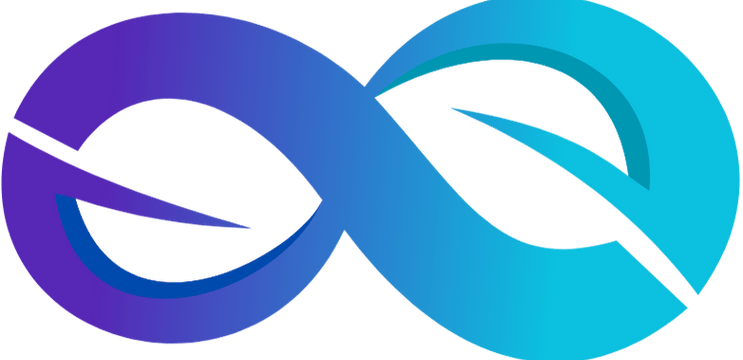
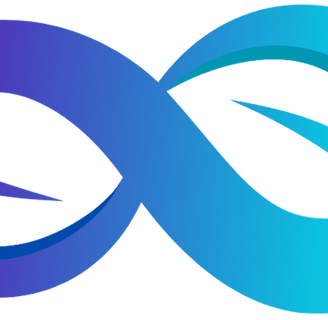
When we hear about Generative AI today, it's hard not to be fascinated by its capabilities: creating lifelike images, composing music, writing stories, designing products, and even developing code. But the path to this point is a fascinating journey filled with breakthroughs, setbacks, and revolutionary ideas. Let’s explore how we got here, from the inception of artificial intelligence to the sophisticated Generative AI models we interact with today.
The Beginnings of AI: Laying the Foundation
The roots of Generative AI can be traced back to the mid-20th century, when artificial intelligence as a concept first emerged. In 1956, a group of scientists gathered at Dartmouth College for what would be known as the Dartmouth Conference, marking the birth of AI as a field of study. Their goal? To explore the possibility of creating machines that could “think” and perform tasks typically associated with human intelligence.
Early AI was rule-based and logic-driven, focused primarily on solving mathematical problems and proving theorems. Despite initial optimism, this rule-based AI was limited, relying heavily on predefined instructions. It wasn’t until the introduction of machine learning—a way for machines to learn from data rather than follow explicit rules—that AI began to exhibit more complex behaviors.
The Rise of Machine Learning and Neural Networks
In the 1980s and 90s, machine learning gained traction, and neural networks (computer systems inspired by the human brain) emerged as a promising approach. However, the technology of the time could not fully support the processing power required for large-scale neural networks. This, coupled with limited access to large datasets, resulted in what’s known as the “AI Winter”—a period when AI research funding and interest waned.
But by the early 2000s, advancements in computing power and access to vast datasets sparked a resurgence. Machine learning algorithms, especially deep learning (a subset of machine learning), started to show impressive results, allowing models to “learn” complex patterns. Deep learning networks with multiple layers (hence “deep”) could now process large amounts of data, enabling breakthroughs in image and speech recognition. This era of deep learning laid the groundwork for Generative AI.
The Dawn of Generative Models: GANs and Transformers
The true inception of modern Generative AI can be credited to the development of Generative Adversarial Networks (GANs) in 2014, introduced by Ian Goodfellow and his team. GANs consist of two competing neural networks: a generator, which creates new data, and a discriminator, which evaluates it. Through this competition, GANs can produce remarkably realistic images, marking a transformative moment in Generative AI’s history. Suddenly, AI could create—whether generating lifelike faces, artworks, or virtual worlds.
While GANs were game-changers, another breakthrough model would soon reshape the field further: the transformer. First introduced by Vaswani et al. in 2017, transformers revolutionized natural language processing (NLP) by enabling AI models to understand and generate language with remarkable accuracy. This model architecture paved the way for large language models (LLMs) like OpenAI’s GPT series, which could generate coherent text, translate languages, and even answer questions.
The Age of Large Language Models (LLMs)
With the development of increasingly powerful LLMs, such as GPT-2 in 2019 and GPT-3 in 2020, AI models could now produce text that was almost indistinguishable from human writing. The GPT (Generative Pre-trained Transformer) models trained on massive datasets captured nuances, slang, and even stylistic choices, opening up possibilities for chatbots, virtual assistants, automated content creation, and beyond.
The significance of GPT-3 and its contemporaries can’t be understated. For the first time, AI wasn’t just responding to instructions but could engage in open-ended, creative tasks. Businesses, educators, and artists quickly began experimenting with these new tools, exploring their potential to reshape industries from marketing to media to software development.
Current Capabilities and Applications of Generative AI
Today, Generative AI has advanced far beyond the capabilities of GANs and early LLMs. Models like GPT-4 and other multimodal models (those capable of processing both text and images) have further expanded the horizons. Applications now range from creating photorealistic images and producing art in specific styles to generating business reports, simulating conversations, designing products, composing music, and even assisting in drug discovery.
Notably, these tools have become increasingly accessible, with interfaces that allow users to leverage complex AI models without needing deep technical expertise. Industries are embedding Generative AI into their workflows, transforming fields like healthcare, education, entertainment, and beyond.
The Challenges and Ethical Implications
However, along with exciting capabilities, Generative AI has also introduced ethical and practical challenges. Issues such as misinformation, copyright infringement, privacy concerns, and job displacement are part of the growing pains. Deepfakes, AI-generated images or videos that manipulate real likenesses, pose risks to privacy and authenticity in media. The potential misuse of these technologies for malicious purposes, such as spreading fake news, also raises serious concerns.
To address these issues, organizations and governments are now working on policies, ethical guidelines, and AI safety research to ensure that Generative AI is developed and used responsibly. Transparency, accountability, and inclusive development are crucial as we move forward to balance innovation with ethical standards.
Looking Ahead: The Future of Generative AI
As we look to the future, the trajectory of Generative AI suggests even more transformative changes. We’re already seeing the beginnings of generative agents—AI that can learn continually and interact autonomously, paving the way for AI that could assist in complex problem-solving or scientific research. With the rise of reinforcement learning and self-improving models, future Generative AI might not just create but adapt and evolve in real-time.
Furthermore, we can expect a stronger emphasis on collaboration between humans and AI, where Generative AI acts as a creative and cognitive partner rather than a mere tool. Fields like medicine, architecture, engineering, and climate science could benefit immensely from such partnerships, enabling humanity to tackle challenges previously thought insurmountable.
Conclusion: A Revolution in the Making
From the early days of rule-based systems to today’s advanced neural networks and large language models (LLMs), the journey of Generative AI reflects the rapid pace of technological innovation and our expanding grasp of creativity, intelligence, and possibility. We’ve reached a moment where AI is not just replicating human tasks but inspiring new avenues of thought, production, and collaboration.
Yet, the next phase of Generative AI’s potential can only be fully realized through frameworks like GenAIOps , which have gained significant momentum across industries. GenAIOps builds on established practices from DevOps, MLOps, and LLMOps, providing the cultural and technical backbone necessary to manage the unique complexities, security considerations, and scalability demands of generative models. By standardizing adaptive processes and promoting best practices, GenAIOps ensures the ethical and efficient operation of generative AI at every stage, from development to deployment.
By embracing frameworks like GenAIOps, we lay the groundwork for a future where Generative AI can augment human potential responsibly, ethically, and sustainably. The journey here has been nothing short of extraordinary—and with the movement of GenAIOps, the true potential of Generative AI is within reach.