The GenAIOps Operating Model
Generative AI presents transformative potential across business functions, from automation to augmentation of creative and strategic workflows. However, the path from concept to operational impact can be unclear. The GenAIOps Operating Model addresses this by offering a structured, end-to-end approach for implementing Generative AI in a way that is aligned, tested, and scalable. It helps organisations move from exploratory efforts to production-ready, sustainable solutions by guiding them through three distinct phases: Strategy, Proof of Concept, and Productionisation.
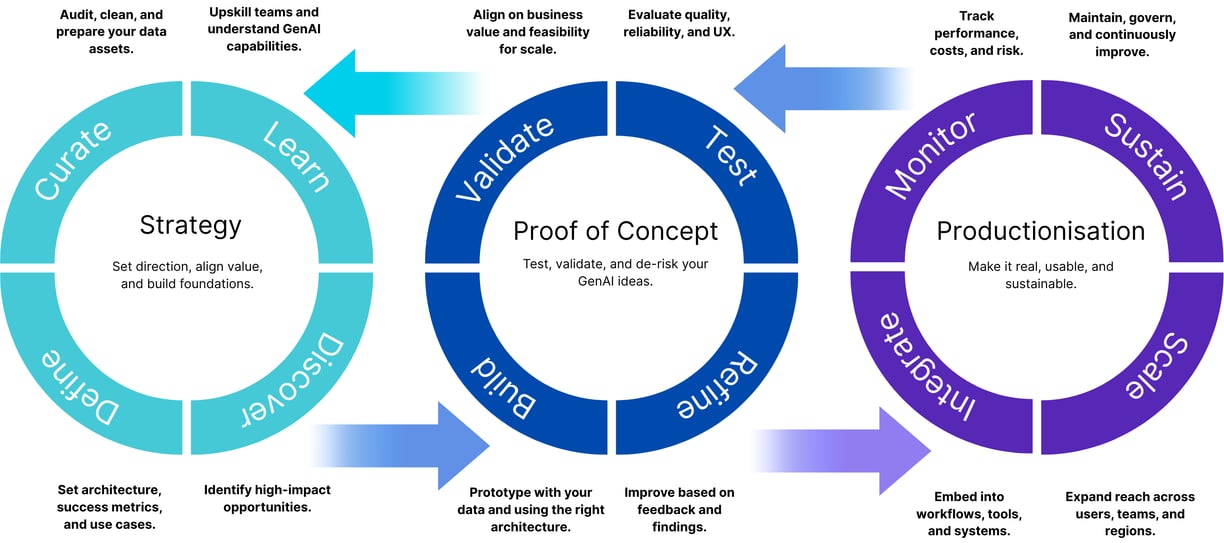
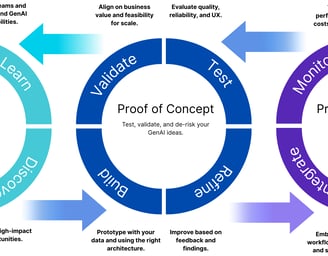
Strategy
The Strategy phase provides the foundation for all Generative AI efforts. Its purpose is to ensure that initiatives are grounded in business relevance, organisational readiness, and clear intent. It enables teams to focus on the right problems, prepare the necessary data, and align stakeholders around common goals before any solution is developed.
Curate
Data is the raw material of Generative AI, and its quality directly determines the success of any initiative. This step begins with a comprehensive inventory of available and applicable data assets, followed by an assessment of their relevance, consistency, completeness, and compliance status. It includes establishing clear data taxonomies, cleaning inconsistent records, addressing gaps, and aligning data with required privacy and governance standards. Equally important is ensuring that your data is easily accessible by technical teams, with secure, structured pipelines for ingestion and transformation. This is the stage where data governance principles should be reinforced to prevent downstream risks.
Learn
You must build foundational knowledge and shared understanding of Generative AI across all levels. This includes awareness training for leadership to ensure informed decision-making, as well as deep technical upskilling for engineers, analysts, and product teams who will be hands-on in implementation. Learning also involves setting up internal forums for knowledge sharing, creating playbooks for Generative AI experimentation, and documenting best practices. This phase should also focus on aligning stakeholders around ethical considerations, risk profiles, and organisational goals related to Generative AI.
Define
This step formalises the approach by narrowing the focus to specific, high-value use cases. It involves creating a clear problem statement, identifying target users, estimating potential impact, and setting measurable success criteria. From a technical standpoint, you can define the architecture, tools, and platforms that will be used to prototype and eventually scale the solution. Platform decisions should consider cost, customisation, control, scalability, interoperability, and integration capabilities, whether using open-source models, commercial APIs, or in-house deployments. Operational readiness is also assessed here, understanding dependencies, assigning responsibilities, and planning timelines. Risk and compliance planning should also be embedded at this stage, evaluating potential legal, ethical, and regulatory implications including data privacy, intellectual property, bias and fairness risks, and requirements for transparency and explainability. Additionally, security and abuse prevention measures, such as access control, prompt injection defences, and content safeguards should be considered. By integrating these considerations into technical and operational design, you can avoid costly rework and ensure their initiatives meet compliance standards from the outset.
Discover
Discovery is a process to identify where Generative AI can deliver the most value. It includes mapping internal pain points, process inefficiencies, and unmet customer needs through collaboration with business and technical stakeholders. The aim is to surface ideas that are not only desirable but also feasible within the your current capabilities. Prioritisation frameworks such as value-effort matrices or strategic alignment filters are applied to identify the most promising opportunities. This step should also surface potential risk exposure and governance challenges that could arise from deploying Generative AI in specific use cases. Early identification of these risks allows mitigation strategies to be baked into design and delivery plans.
Proof of Concept
In this phase, selected ideas are tested and refined in a controlled environment to reduce uncertainty, validate assumptions, and assess the practicality of Generative AI solutions. It ensures only viable, valuable concepts proceed to production.
Build
This step involves creating a functional prototype that demonstrates the core capabilities of the Generative AI solution. The build should be lightweight but operational, designed to test key hypotheses rather than deliver full functionality. Data pipelines are connected, model architectures selected, and interfaces developed to the minimum extent necessary for evaluation. Code and infrastructure should be modular and loosely coupled to allow for fast iteration. A well-built prototype accelerates feedback loops and brings abstract concepts into practical focus.
Refine
Once the prototype is in use, feedback is collected from your stakeholders, end users, and system performance metrics. The refinement process focuses on improving model outputs, enhancing interaction flows, and addressing technical limitations identified during early use. Adjustments may include fine-tuning model parameters, improving data preprocessing steps, or restructuring user interfaces. Refinement cycles are short and frequent, emphasising speed and learning over perfection. This step ensures that the evolving solution aligns closely with user needs and technical expectations.
Test
Testing is comprehensive and rigorous, encompassing both technical performance and user experience. It includes validation of model accuracy, latency, security, reliability, and scalability. Edge cases and failure scenarios are intentionally introduced to assess system resilience. User feedback is formally captured through usability tests, structured walkthroughs, and analytics. Test environments should mirror production conditions as closely as possible, with synthetic or sandboxed data to simulate realistic behaviour. Security and misuse scenarios, such as prompt injections or adversarial inputs should also be evaluated to harden the solution. This step ensures the prototype is ready for critical evaluation and informed decision-making.
Validate
The final activity in this phase is to determine whether the solution is ready to move forward. Validation looks at the solution holistically; technical feasibility, operational impact, user adoption potential, and alignment with regulatory and organisational constraints. It includes a go/no-go decision based on predefined criteria such as performance benchmarks, stakeholder endorsement, and resource readiness. Documentation is updated to reflect lessons learned, remaining risks, and suggested next steps. Validation acts as the quality gate between experimentation and production.
Productionisation
Once validated, the focus shifts to embedding the solution within your organisation, scaling it across relevant teams, and maintaining its performance over time. This phase transforms a promising prototype into a durable, operational asset.
Integrate
Integration is the process of embedding the Generative AI solution into day-to-day workflows, applications, and systems. It includes configuring APIs, automating inputs and outputs, setting permissions, and ensuring seamless interaction with adjacent platforms. Business processes are updated to accommodate the solution, with documentation and training rolled out to affected users. Integration also involves aligning performance monitoring and support protocols with existing IT operations. User transparency is critical, users interacting with Generative AI systems should be clearly informed that they are doing so, especially in customer-facing or decision-support contexts. Business continuity planning, including clear fallback processes and escalation paths, must also be addressed to ensure operational stability in case of system failures or unintended behaviours.
Scale
Scaling ensures that the solution can serve a broader user base and handle increased load without degradation in performance or user experience. It requires evaluating infrastructure capacity, automating deployment processes, and establishing enterprise-wide access controls. Scaling also involves extending training, onboarding, and support services to accommodate new users. Governance models and compliance checks are scaled in parallel to maintain consistency and control. Effective scaling also requires clear change management planning, communicating shifts in workflows, preparing stakeholders for transition, and ensuring adoption by integrating change enablement into rollout plans. The goal is to increase reach and impact while maintaining stability and control.
Sustain
To remain effective, the solution must be maintained and evolved whilst remaining cost effective and environmentally conscious. Sustaining includes regular updates to data pipelines, model retraining, performance optimisation, and user experience improvements. A structured support model is put in place, including tiered issue resolution, documentation updates, and a roadmap for enhancements. Governance boards and audit logs ensure transparency and accountability. Sustainability also includes clearly defined ownership models, with responsibilities assigned across engineering, operations, product, and compliance teams to ensure accountability throughout the lifecycle. Lifecycle practices such as model versioning, rollback strategies, deprecation planning, and periodic revalidation help ensure the system remains current, safe, and aligned to business needs.
Monitor
Continuous monitoring is essential for ensuring operational health, risk mitigation, and ongoing improvement. Monitoring involves tracking usage metrics, system performance, model drift, error rates, and user satisfaction. Dashboards and alerts are configured to provide real-time insights, and periodic reviews are conducted to assess alignment with business goals. Monitoring should also include feedback loops with end users and business stakeholders to surface areas for continuous refinement. It is tightly integrated with incident response and change management processes, with predefined escalation and rollback mechanisms in place to respond to critical issues. This final step ensures the solution is not only functional but also reliable, compliant, and strategically aligned over time.
Bringing It All Together
By following a structured, end-to-end approach to Generative AI implementation, organisations can move beyond experimentation and deliver meaningful, scalable impact. The GenAIOps Operating Model brings clarity, control, and confidence to each stage of the journey from planning and prototyping to integration and long-term performance. With the right foundations, oversight, and adaptability in place, Generative becomes a catalyst for continuous innovation and strategic advantage.