Why GenAIOps?
The future of software is agents but how do we create, train, test, control, evaluate and understand them? The answer is collabration, shared best-practice and continuous improvement. In short, the answer is GenAIOps.
Glen Robinson
12/7/20236 min read
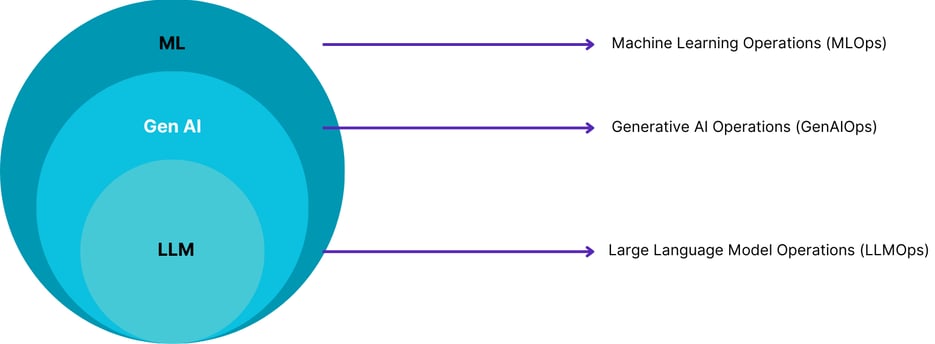
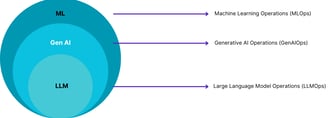
An Agent-led future
In November 2023, Bill Gates wrote, “Agents are not only going to change how everyone interacts with computers. They’re also going to upend the software industry, bringing about the biggest revolution in computing since we went from typing commands to tapping on icons.”(GatesNotes - Nov 2023: https://www.gatesnotes.com/AI-agents)
That is quite a statement coming from someone who has been predicting and delivering the future for over 30 years.
He is describing a world where technology can interact with humans just as we have been watching in sci-fi movies for the last 50 years. When Tony Stark speaks to Jarvis, he sends it verbal instructions and off it goes to do something complex; aggregates a bunch of data and comes back with a curated information, or takes an action, or requests someone/something else to do something. In this example, Jarvis is an agent, and Bill sees a world in the next 5 years where we will all be doing the same.
For some, this may still sound like sci-fi, but the reality is that the future is already here.
Organisations today are just starting to get to grips with generative AI (GenAI) and encountering all manner of new opportunities and risks, and yet this is just an enabler for something even bigger on the horizon. Agents.
GenAI is trained on data and has learnt to understand language in a way that in some regards, has now surpassed the abilities of most humans. Developers can connect GenAI models to data sets which allows it to reason over the data and come back with insights, summarisations, sentiment analysis, as well as use it as a basis for the creation of original content.
An agent takes an input, say a conversational voice command from a user, and then orchestrates a series of connected data sources and services to produce an outcome. An agent is a one-stop shop from a user interface perspective and it will abstract away the complexity of needing to use specific applications for specific tasks or accessing specific sets of data to understand something. It is the agent's job to do this all for you.
Today, technically, this is possible. A GenAI interface can understand the nature of a question being asked and connect to appropriately connected systems via an orchestrator, which gathers information from various data sources, executes necessary processing using digital services, reviews the responses, revisits more systems if needed, until eventually it has what it needs to curate and provide a response to the user.
The case for GenAIOps
The shift we are witnessing today driven by the mass adoption of GenAI is only the start of what is to come. When the mass consumption of cloud services started, there were challenges and opportunities that inspired the near universal adoption of DevOps. Things had to be done differently and DevOps helped developers use agile methods to create cloud-based applications.
The same is happening with GenAI. The mass development and adoption of agents has major challenges to overcome. The “transparency deficit” of LLMs (large language models), the undetected biases existent in models trained on web content, the ethics and law of copyright, the benchmarking of agent performance, the definition of tasks that need humans in the loop, the metrics that allow us to take humans out of the loop, the aggregation effect of multi-agent environments - and a million other issues - all need to be resolved quickly and collaboratively.
For this reason, the global Gen AI community is starting to develop GenAIOps. If we get it working and adopted, it will help not just the developers, but the whole business create GenAI powered agents that are transparent, trustworthy, economical, and provide end user value.
GenAIOps is a model that encompassed the entire GenAI lifecycle and accounts for the issues particular to GenAI like ethics, bias and LLM management. From initial model selection, all the way to validating the responses generated by the model, and everything in-between, GenAIOps will unify the development of agents with their operation and will have to develop at the same pace as the underlying technology. For this reason, a whole-system approach to automation, monitoring and cross-functional alignment will be crucial through the GenAI lifecyle.
In a recent paper (Mastering LLM Techniques: LLMOps, 15 Nov 2023), Nvidia wrote, “GenAIOps extends MLOps to develop and operationalise generative AI solutions. The distinct character of GenAIOps is the management of and interaction with a foundation model.” They also offered the diagram above which demonstrates how it fits within the wider ML (Machine Learning) eco-system.
Ultimately, GenAIOps will be all about bringing together teams that have never had to cooperate in the way that the agent-driven future will force them to cooperate.
Stakeholder roles in an age of GenAIOps
There are now multiple groups of stakeholders who have a personal stake in the design and consumption of GenAI systems.
The GRC community. Governance, risk, and compliance help manage organisational risks. These risks come in many forms such as brand and reputation, security, copywrite infringement, IP (Intellectual Property) protection, and compliance. GenAI can expose any organisation to these risks at many stages of the GenAI lifecycle, from the data being used to train the model, to the responses it provides. These risks are also not static and the context around data can change quickly; something could be classed as sensitive one minute, but then not the next. GenAI will want to account for these changes rapidly, and the business will need to ensure appropriate guardrails are being followed.
The executive community. These individuals will be focused in two primary areas. The use of GenAI to drive business efficiency, and the creation of net new value. They will need metrics that demonstrate the ROI (Return on Investment) of the costs of any GenAI system and the efficiency it creates, or the new opportunities it presents. Therefore, detailed cost metrics as well as options to drive optimisations in the GenAI system will be key to make informed business decisions.
Increasingly organisations, and therefore the executives within, are making more environmentally sustainable decisions. Clarity over the carbon emissions generated through the consumption and training of a model will be considered. Trade-offs with small language models (SLM’s) vs large language models (LLM’s) will need to be made to achieve sustainability targets.
The developer community. Developers will need to build and operate the GenAI systems and will require similar capabilities to their DevOps tooling and will need a mature set of data tooling along with tools that allow them to align the GenAI systems to the business strategy and values.
Testing of the models will have to consider the ability of the GenAI system to take into account the organisations values around ethics, the environment, and the risk appetite. Any one of these areas could change over time and systems and processes need to be in place to make adjustments and provide assurances that the technology is achieving organisational goals.
Transparency & Control
The importance of organisations understanding the fundamentals of GenAIOps cannot be overstated. Many applications in the future will have GenAI as a component part and as we evolve to more agent-based designs, these GenAI systems will be making choices on the data and services to use, based on criteria set by the app developer or the end user of the agent. The agent will then orchestrate a complex series of processes, taking this context into consideration to produce a result.
If you are a data owner or service owner, it will be your responsibility to ensure you can clearly describe the characteristics of your service, such as the economics, the ethical considerations, the sustainability metrics, so that agents can make decisions on whether to use your service or data, or not.
Any approach to GenAI that does not deliver transparency and control across the full lifecycle of agents both individually and collectively, will not survive the challenges of enterprise deployment.
The Centre for GenAIOps
On a personal level, I am excited about our future with GenAI agents. I am an advocate for all the good they will drive for both humanity and our natural world. So rather than see implementation in the early months stumble as organisations try to coalesce around best-practice, I have teamed-up with other industry leaders and founded The Centre for GenAIOps.
It is a not-for-profit Community Interest Company with the mission of accelerating the development and adoption of best practice in this space. No one person or organisation has all the answers. If you are reading this, then you have a part to play as we try to access all the benefits of GenAI safely and rapidly. Please comment, share, or contact me with any thoughts you have or ways you can support us.