Navigating the Future with GenAIOps: Core Principles and Frameworks
Starting to examine the how and why of GenAIOps
Harrison Kirby & Glen Robinson
11/15/20232 min read
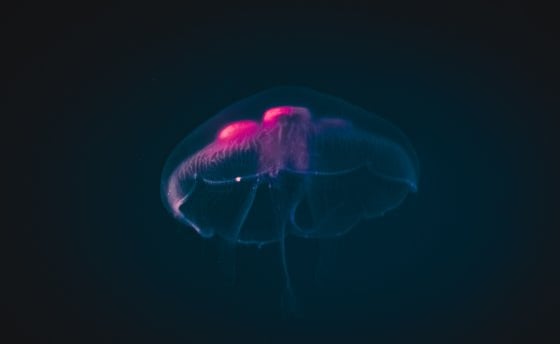
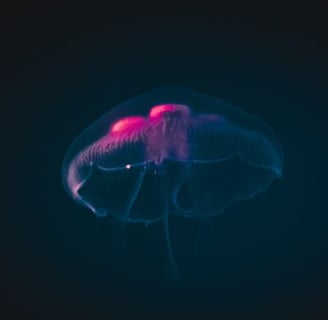
Introduction
As businesses increasingly turn to generative AI to drive innovation and solve complex challenges, the principles and frameworks governing its use become critical. GenAIOps, which extends the agile and integrative spirit of DevOps into the realm of generative AI, provides a roadmap for this journey. In this article, we delve into the core principles and philosophies of GenAIOps, explore how to build a GenAIOps framework, and contrast GenAIOps with traditional DevOps.
Core Principles and Philosophies of GenAIOps
GenAIOps is underpinned by a set of guiding principles and philosophies that distinguish it from other technology practices:
1. Collaboration and Integration: At its heart, GenAIOps fosters a culture of collaboration among data scientists, AI ethicists, IT professionals, and business stakeholders. This interdisciplinary approach ensures that generative AI solutions are developed in alignment with business goals and ethical standards.
2. Continuous Learning and Adaptation: Generative AI is about learning from data and evolving. Similarly, GenAIOps emphasizes continuous learning and adaptation of AI models and practices to keep pace with business and technological changes.
3. Scalability and Reliability: Ensuring that AI solutions can scale effectively and maintain reliability across diverse enterprise environments is a cornerstone of GenAIOps.
4. Ethical AI and Compliance: GenAIOps places a strong emphasis on developing AI solutions that are ethical and comply with regulations, recognizing the growing importance of responsible AI in the business world.
5. Operational Excellence and Efficiency: Streamlining operations to achieve efficiency without compromising on the quality or ethics of AI solutions is a key principle of GenAIOps.
Building a GenAIOps Framework
Developing a GenAIOps framework involves several key steps:
1. Assessment and Planning: Understand the current capabilities and needs of the organization in terms of generative AI. This includes assessing the technical infrastructure, skill sets available, and business objectives.
2. Tool and Technology Selection: Choose the right set of tools and technologies that align with the specific needs of generative AI applications, considering factors like scalability, integration capabilities, and support for continuous learning.
3. Process Integration: Seamlessly integrate generative AI processes into existing business and IT workflows, ensuring that the transition to GenAIOps is smooth and adds value to the existing processes.
4. Monitoring and Governance: Establish robust monitoring and governance mechanisms to ensure the ongoing effectiveness, compliance, and ethical alignment of AI solutions.
5. Feedback and Iteration: Create feedback loops to continuously gather insights from AI operations and use this feedback to iteratively improve AI models and practices.
GenAIOps vs. Traditional DevOps
While GenAIOps builds upon the foundation of DevOps, there are distinct differences:
1. Focus Area: Traditional DevOps focuses on software development and IT operations, whereas GenAIOps extends this to encompass the entire lifecycle of generative AI in enterprise settings.
2. Interdisciplinary Integration: GenAIOps involves a broader spectrum of stakeholders, including AI ethics experts and data scientists, in addition to IT and development teams.
3. Complexity and Scope: The complexity in GenAIOps is inherently higher due to the nature of AI models and the need for continuous data analysis and learning.
4. Ethical and Regulatory Considerations: GenAIOps places a greater emphasis on ethical considerations and regulatory compliance specific to AI.
Conclusion
GenAIOps represents a paradigm shift in the way enterprises approach generative AI. By adhering to its core principles and carefully constructing a framework that supports these ideals, organizations can harness the transformative power of AI while ensuring ethical, efficient, and effective operations. As we move further into an AI-driven era, GenAIOps stands as a critical enabler of sustainable and responsible technological advancement.